About Me
I am currently on the progress of Master’s degree at Seoul National University, supervised under Prof. Jungwoo Lee. My area of research is statistical machine learning and inference, with a focus on estimating uncertainty. My reseach topic also focuses on applying uncertainty to other tasks such as computer vision or reinforcement learning. CV is available at here.
Publications / Preprints
We propose a new Class Activation Map (CAM) method which achieves state-of-the-art in a weakly supervised object localization problem. The proposed method utilizes uncertainty obtained from dropout layers. Our method can be applied in a pre-trained classification network without additional training if it contains dropout in fully connected layers.
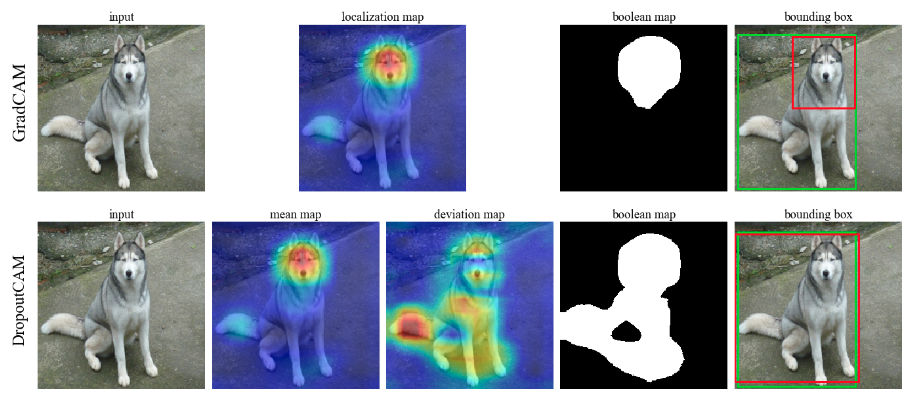
We study the robustness to the geometric transformations in a specific condition where the black-box image classifier is given. We propose an additional learner, REST, that transforms the warped input data into samples regarded as in-distribution by the black-box models. Our work aims to improve the robustness by adding a REST module in front of any black boxes and training only the REST module without retraining the original black box model in an end-to-end manner.
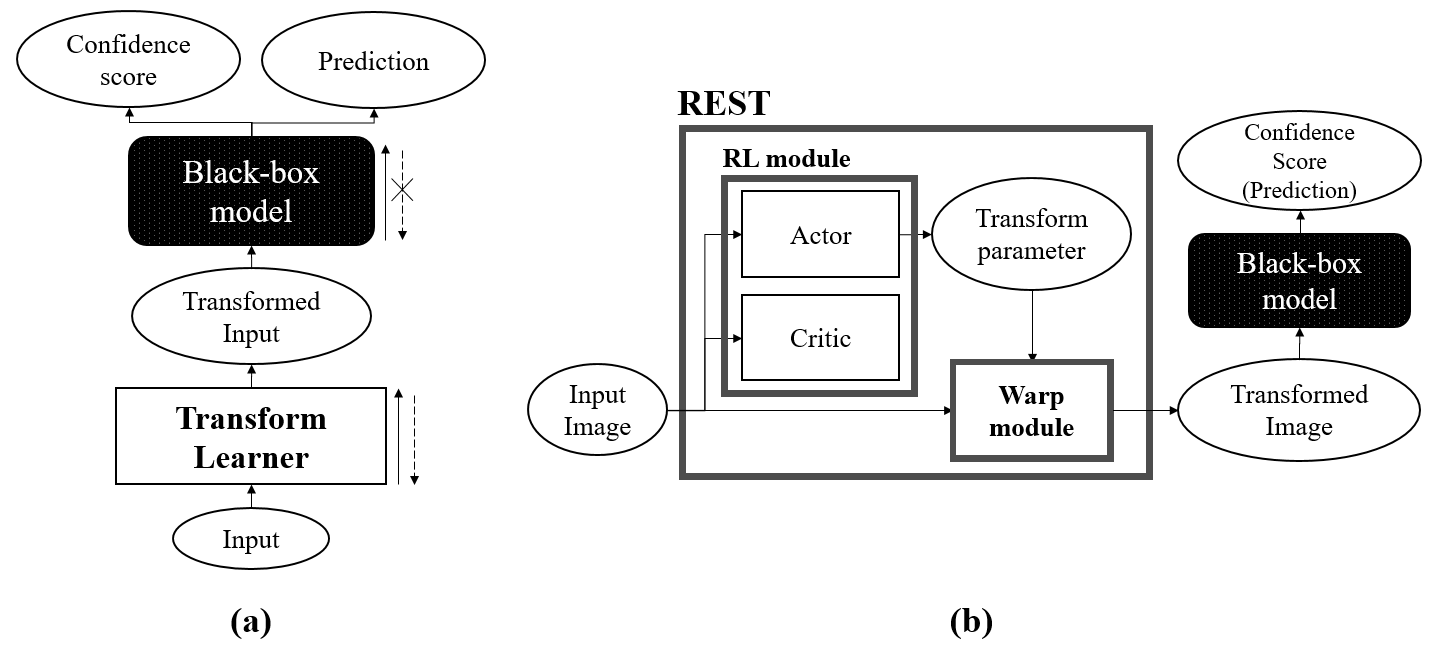
Quantitative susceptibility mapping (QSM) is a quantitative approach for measuring magnetic susceptibility using MRI. Recently, deep neural network-powered QSM, QSMnet, successfully performed ill-conditioned dipole inversion in QSM and generated high-quality susceptibility maps. However, QSMnet underestimates susceptibility in hemorrhagic lesions. We overcome this limitation by data augmentation method to generalize the network for a wider range of susceptibility.
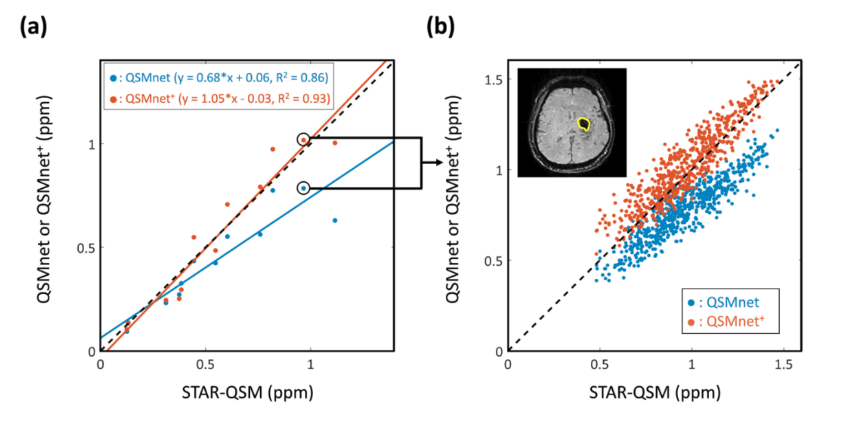
We show that predictive uncertainty can be efficiently estimated when we incorporate the concept of gradients uncertainty into posterior sampling.
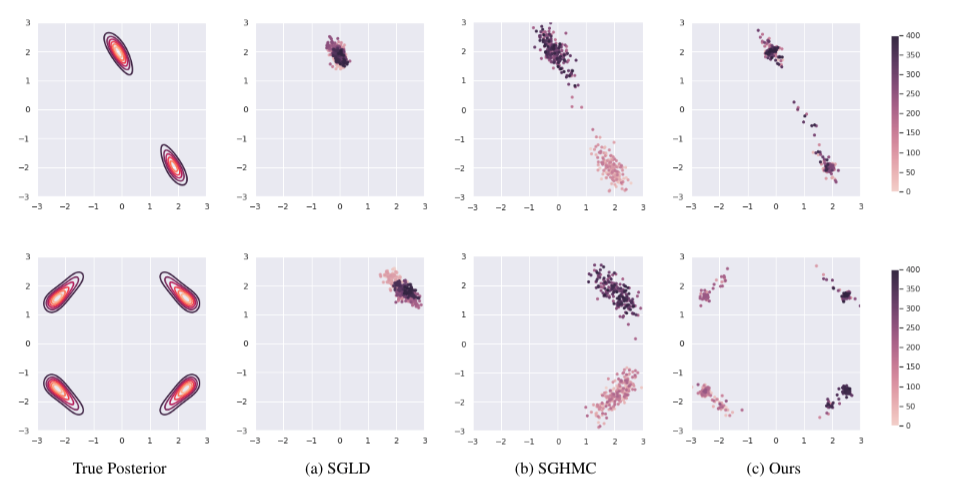